A/B tests developer’s manual
A 11 minutes story written on Sep 2017 by Adrian B.G.
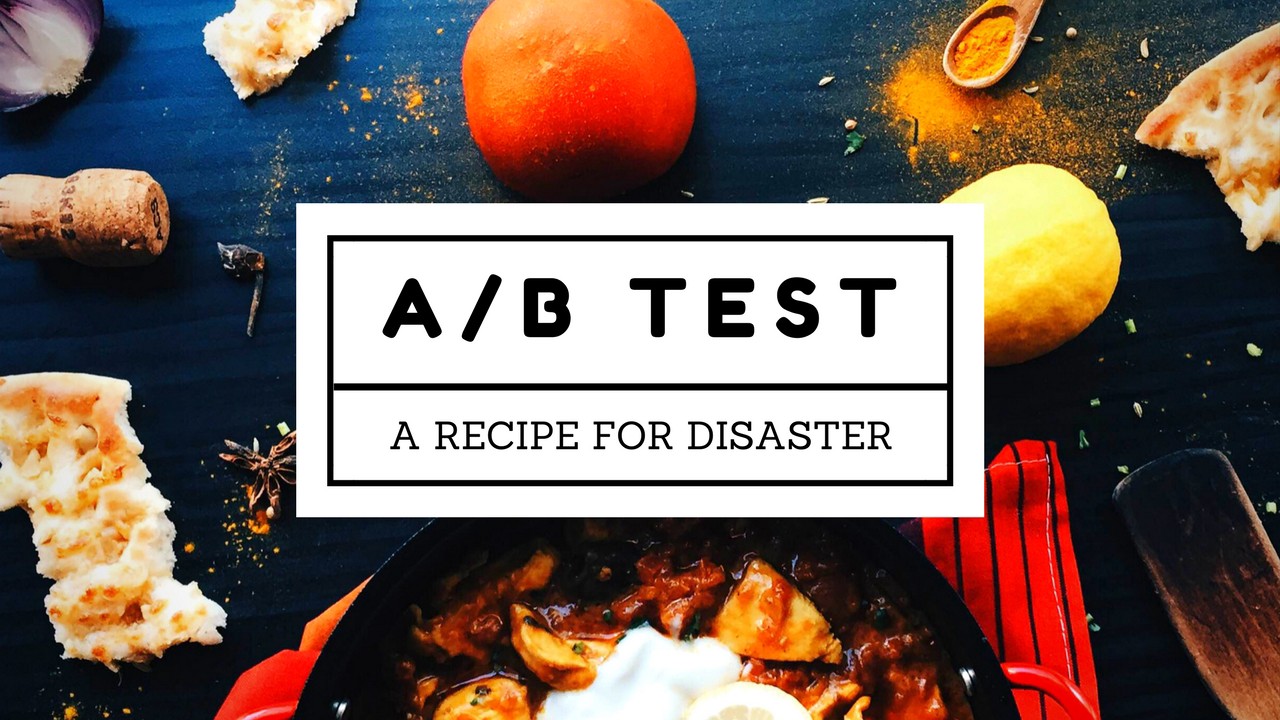
THis article is meant to be a comprehensive guide for web developers that found themselves in:
Best case scenario: Your [product owner,boss,producer] found out about A/B tests and you are here to learn more about how to implement them.
Worst case scenario: Your product is already a mess because of A/B tests, and you want to clean it up.
Either way, I’m writing this article so you do not have to repeat our mistakes.
In the last 5 years I worked mostly in the gaming industry. I had to implement hundreds of A/B tests and I learned it’s a powerful 💪🏾 tool. In the same time I learned that if you do not pay enough attention, your code transforms in a spaghetti 🍝restaurant.
I wish there would be a single simple 🎯way to implement A/B tests without making a mess in your code,but I don’t know any. By definition your code needs to have multiple versions of the same behavior.
Intro ⚓
Skip this block if you are already familiar with A/B testing.
A/B test is also called multivariate testing, A/B/C/D testing, split testing or bucket testing. It is an iterative process of experimentation, that helps you find out what is better for your product. More formal definitions: [here] (https://www.optimizely.com/ab-testing/) and here.
Your product (game, app, website, shop …) can grow in 2 ways:
- a person says “feature X will improve the Y KPI by 30%”, and you implement this feature. We, the mortal humans cannot predict the future, we can only guess it.
- a person says “this X feature is the best”, other says “Y feature is the best”. You implement X,Y,Z variants and measure exactly which one is better.It may be none, one or more of them. You keep the best versions and improve them with further split tests.
The tests are done on smaller, but representative samples of users. This way you can test multiple versions in parallel and mitigate the effects. You do not know how it will affect the user behavior, so you want to minimize a possible damage.
You start by distributing your users into buckets, each bucket will provide a different user experience and you collect the data and analyze the impact of each version. You choose the best bucket and roll-out it to all the users. The process is more complex than this, but this was the main idea.
Basic example: you want to find out the price point of a new product you can make a test. 50% of the users are left out of the test, with $5 price. The remaining 50% are split equally into 5 A/B test versions ($5 control group, $10 version 1, $15 version 2, $20 version 3, $25 version 4).
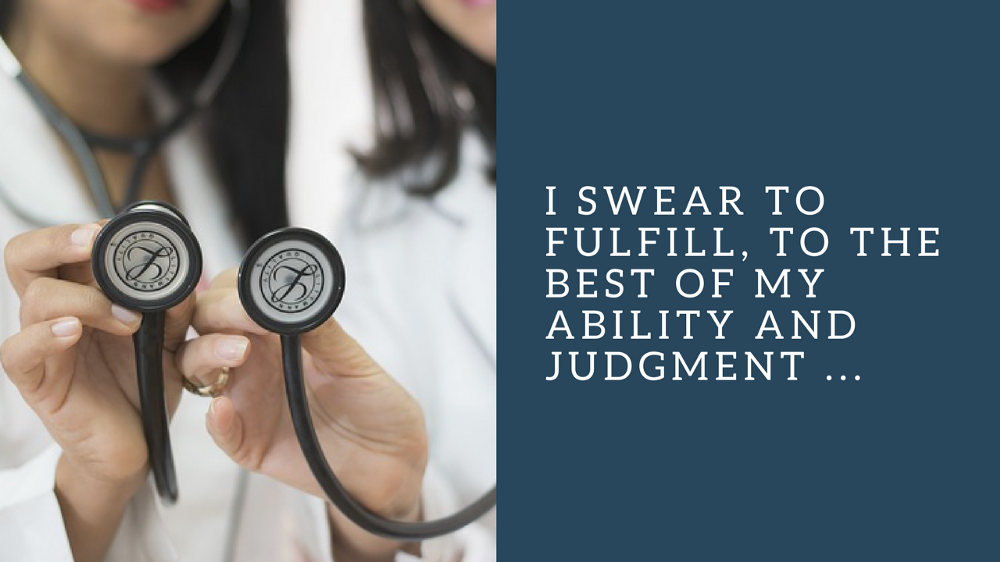
Professional commitment ✍
I, the developer, swear not to be biased. We cannot allow any personal/technical difference or issue to affect the A/B test result (user behaviors)for example:
- loading— make sure all the resources are loaded from the same source (CDN/hosting), so the network times are similar for the users
- size— make sure the file sizes are the same for all the versions, 1 button has 1mb background and the rest are 50kb
- …you get the picture. All the users must start from the same premise. If a technical irregularity appears please let the team know and repeat the test.
We, the developers are in charge of the implementation and technical details, we must guarantee the technical unbiased and act as a firewall.
I, the developer, swear to collect the right data from the users and do not mess with the tracking. Easy to say, hard to do. The main idea is that the A/B test result is based on the KPI events, by observing users behavior during the split test. Data anomalies can mean “bugs” or a clear “winner”.
Usually when something is too good to be real, it is a bug.
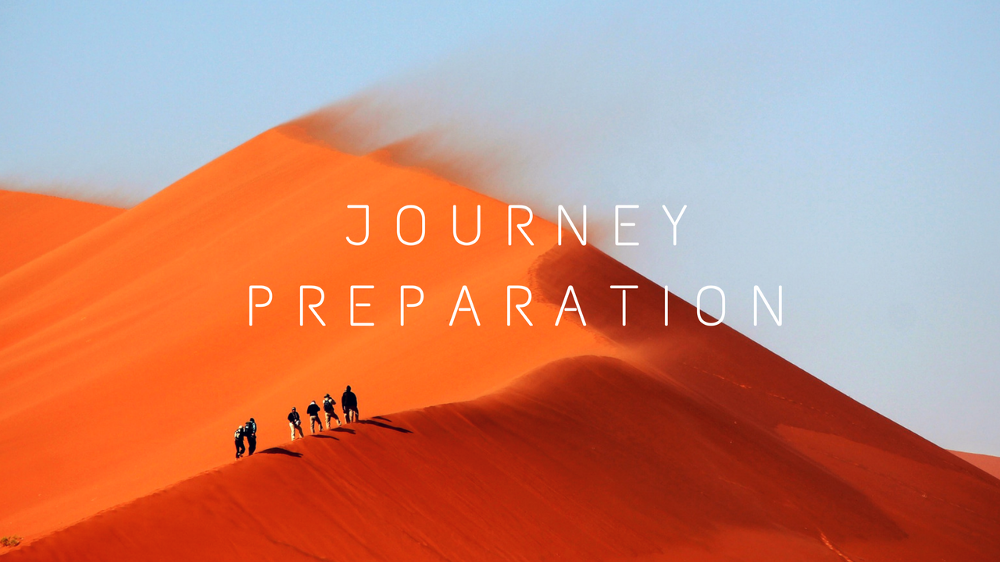
A. Good to have 🛠
It’s easier to work with a better codebase. If your code is already a mess, the A/B tests will multiply it.
Modules If the code is already split to modules (modules, files, classes) most likely you will only need to modify 1 portion of your code, if not … then you must like spaghetti.
Parameters and configs. Everything in an A/B test is reduced to a parameter value, usually a string value. If your business logic has already the tested parameter as a variable or config value its very easy to implement the test.
No magic values If the parameter you are testing is hard coded in multiple places let this be your learning, do not use magic values (magic numbers are the most common mistake).
No constants. Business logic is not like mathematics or religion. There are no absolute truths.
Constants and magic numbers make unit testing more difficult and A/B testing impossible. I developed a new habit along the years, I refactor all the constant values into variables/parameters or class members.
Composition over inheritance. Replacing just 1 member of a class reduces the A/B test intrusion in your code
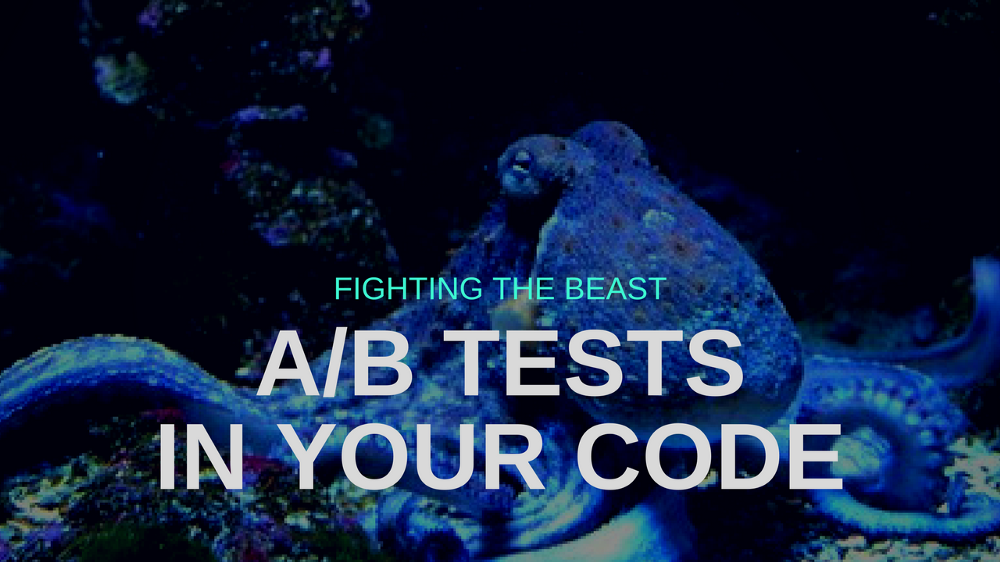
Multivariant test with multiple resources, in your code
B. Implementing A/B tests 👩🏽🏭
A/B test framework. First you need an A/B test service, framework or library. **** If you try to do this in-house (do you really want to reinvent the wheel🎡 and ignore years of research specialized services have?), reduce everything to a simple command, usually it is something like this:
//calling the module ABTest should do everything: put the user in an
//ABTest, read the parameters if any, apply all the logic
$testedValue = AbTest($resourceName,$userId,$defaultValue);
//use the tested value
In 99% of the cases you do not need to know if a user is in an A/B test, you just want the parameter value. The 1% is the tracking events/KPI’s and anti patterns.
Default value. It’s easier to implement an A/B test on a parameter that is used by ALL your users, simpler code means fewer bugs, the effect is the same as using the “null object” design pattern).
//BAD code, prone to bugs
$testedValue = AbTest(resourceName, userId, defaultValue);
if $testedValue == null {
//the user must not see this feature
} else {
//use the tested value
}
Octopus A/B test. A/B tests usually (and by definition) test 1 single thing. Sometimes that thing affects more parts of the project, so you will have to modify 2-n block codes to implement the A/B test. These are harder to implement and maintain, so if you can avoid them do it.
Design patterns toolbox. There are a few design patterns that could be applied in most cases, to mitigate the code mess.
- Null object — as default parameter value
- Creational design patterns(Factory, Builder) when 1 version == 1 class
Small footprint 👣Keep the smallest possible footprint when implementing A/B tests. Best case scenario is when your product owners will want more&more tests. It always starts with 1 “small test”, next thing you realize there are 10 tests with 42 versions, some with the same parameter and already haveing merge conflicts. Also see E.Cleanup and B. — A/B test framework.
1 user 1 abtest 1⃣ This rule is broken in real life scenarios (having too few users, testing different unrelated resources, etc). Keep in mind that tests and user dependencies, complex rules and behaviors will develop later, in the product lifetime, sometimes even worse with concurrent A/B tests.
1 test n parameters. Some more complex A/B tests can be multi parameter, and affects more than 1 resource. Take this into account when choosing the A/B test framework. Ex: background color + header background.
Automatic Tests. By changing the code behavior you may need to mock up the ABTest framework and change your current integration and unit tests.
Offline & multiple platforms. Keep these in mind when tackling A/B tests. Cache your resource values, the user must have a consistent experience!
Panic button. Always keep a feature flag admin somewhere, if something goes wrong you must react fast and disable the A/B test effects.
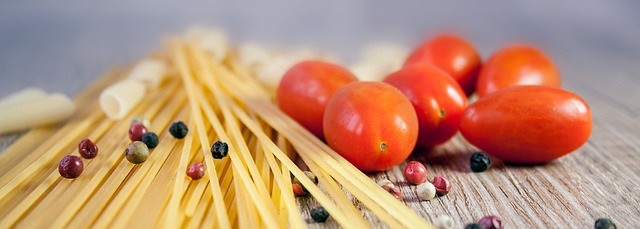
C. Conditions, buckets and flow mess 😱
You solved the previous problems, you learn more about A/B tests and you released a few of them. Now things got more complicated and the producers ask for more specific tests.
$testedValue = "green";
if ($userIsFromAmerica) { //do this ab tests }
else if ($userLifeTime > 10) { //do this tests }
if ($userHasGreenEyes) { //do a new test }
Spaghetti A/B tests. If this code looks familiar you’re already into a black hole. You can blame the producers of course but this does not solve the mess. A good solution I found on the battle field is to encapsulate all this business logic in the ABTest module, especially if the tests have inter-dependencies or rules (if the user is in these split tests, do not put him in those split tests). I know that it is an anti-pattern, you will have a multi-point of change but I think it is the lesser evil. The problem can also be mitigated with a good A/B test platform, that can handle these crazy intricate business rules.
Same experience We should implement the A/B tests logic before the user reaches the tested resource. Example for an website do the A/B tests logic at the login. A bad and faulty experience would be:
- user opens the page, and sees the default Green button
- after the page is loaded the user is distributed in the Red button version
- Two things can happen now, and the wrong was already done. You can refresh the button so the user sees the correct version (Red button), but the user is now biased. If you do not update the button the A/B test is useless, he never saw the Red button but his activity is tracked in the Red Button version KPI’s
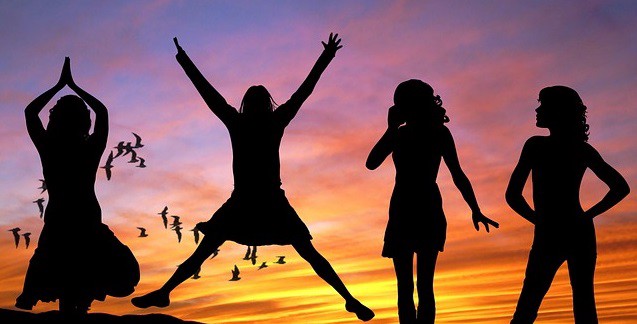
Similar users Most of the time it is not our job, as developers, to select the user pool (it’s done from the A/B admin) but we should keep this in mind when writing the code. There are some common criteria that the product owners use when targeting the audience, to make the test more accurate:
- same lifetime users — new users or with X months since registration
- lifetime value — users that bought X amount of stuff
- same country, same source (referral) etc.
- common action (made X action in the last Y days). Good luck on this one.
Meta strategies. I know that on mobile you can ship different app builds based on A/B tests, countries or other criteria. How to tackle this issue is out of the scope of this article.
As you can see, a developer can ruin the A/B tests results in many ways, with great power comes big responsibility‼
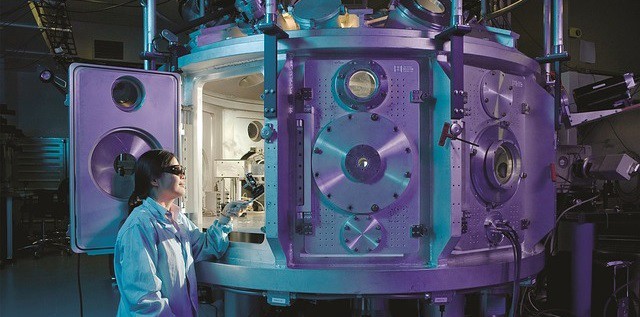
D. Testing 👷🏿♂️
Most likely you have (and want) to implement the ability of a user to switch to any (or exit) A/B test version, for debugging & testing reasons. I know this breaks the A/B test definition, but if your registration process lasts a few minutes, it will save hours to the Q&A department by using this feature.
Do not hard code. Q&A needs flexibility, parameter everything (configs, URL parameters, database flags).
Reset the cache. Do not forget to reset the cache when something changes.
Web tip: we did this technique and it helped us a lot! In the development staging, any visitor could append to the URL ?abtestname=2
to temporarily enter/see the 2nd version, overriding it’s persistent version.
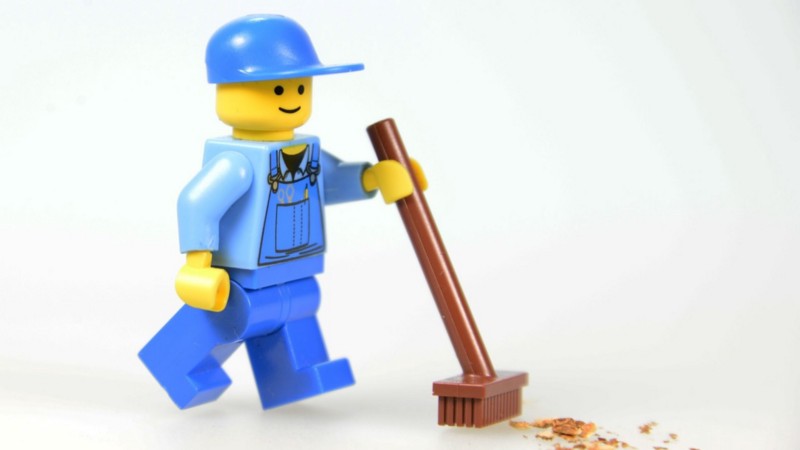
E. Cleanup A/B tests 🛀🏾
Take time to clean your code. Your implementation time estimation and plan should include an after cleaning stage. It’s usually very fast to do, just remove the code that is not used anymore and rollback if needed.
Not cleaning the code will result in a messy, full of noise code base. I can tell you from a first hand experience it is not a #happycode. This is the most common issue I encountered when working under fire.
Remove the resources. Remove the images, sounds, scripts, whatever you added in the project for the A/B test.
Trust me, dozens of tests will follow this one, there is no “I will this clean later, when I have the time in a lighter sprint”.
Remove the leftovers Sometimes, the product owners want some versions to remain in production, even if the tests are closed (because business logic). Fight these decisions, the code is your duty, and the code suffers.
Reversed refactoring. If you had to refactor the code to implement the A/B test (apply a design pattern), this is the time to rollback the code. You do not need the extra logic anymore.
Next ⏩
Usually when the product owner has the results of the first test they want to continue with another one by keeping 1 or more versions and make a new test.
For example after you test five colors of a button you find out that the bright colors have more engagement in the second test you keep on the bright colors and add a few nuances
Takeaways 🐳
- write clean code
- build a panic button (for business & tech reasons)
- make it easy to test/Q&A
- delete the A/B tests code ASAP